Evaluation of AI models for accuracy in the prediction of film thickness in polydisperse polystyrene thin-films
Abstract
Spin-coated polystyrene (PS) thin-films have many applications in the chip manufacturing industry, whose properties vary greatly based on film thickness. A previous study by Wang et al. used a curve-fit machine learning model to create a 3D manifold relating molecular weight (M), polymer concentration in solution, and film thickness of spin-coated monodisperse PS samples [1]. Hence, this study aims to evaluate its effectiveness in predicting polydisperse PS film thickness. We simulated polydispersity by creating bidisperse solutions of PS with ratios of high and low M, M1 and M2, respectively, at varying concentrations. After spin-coating these samples onto silicon wafers [1,0,0], we determined film thickness through ellipsometry. For a given ratio and concentration, we found that the model used by Wang et al. consistently predicted lower thicknesses than actual experimental values. We were able to relate thickness, concentration, ratio M1:M2, and difference between M1 and M2 using a neural network trained on bidisperse mixtures, holding M1 constant at 30K. We compared our models predictions to those of the curve-fit model used by Wang et al. to determine the effects of polydispersity on film thickness. Our data agrees with the theory of constraint release (CR) induced dynamic tube dilation (DTD) for bidisperse mixtures with a wide separation of M1 and M2, which is further corroborated by the viscoelastic data on bidisperse PS solutions from Watanabe et al [2]. Our model coarse-grains the complex molecular dynamics of polymer chains into a parameterized model that inputs concentration, ratio, and difference to predict thickness. Thus, our model provides a foothold into predicting the thickness of multidisperse thin-films by converting a problem of exponentially scaling complexity into linear additivity of bidisperse thicknesses predicted by our model. Further data is needed to refine the model for a broader range of molecular weights, as well as to test its applicability to tridisperse and multidisperse systems.
[1] Wang, A.C., et al. MRS Communications 14, 230–236 (2024).
[2] Watanabe, H. (1999). Progress in Polymer Science, 24(7), 1253-1403.
Conferences, Distinctions, and Publications
See our abstract and slides from our presentation at the Garcia Research Scholars symposium.
Yearbook for the Garcia Scholars Program. See our abstract on pages 67.
Presentation from the end-of-program symposium.
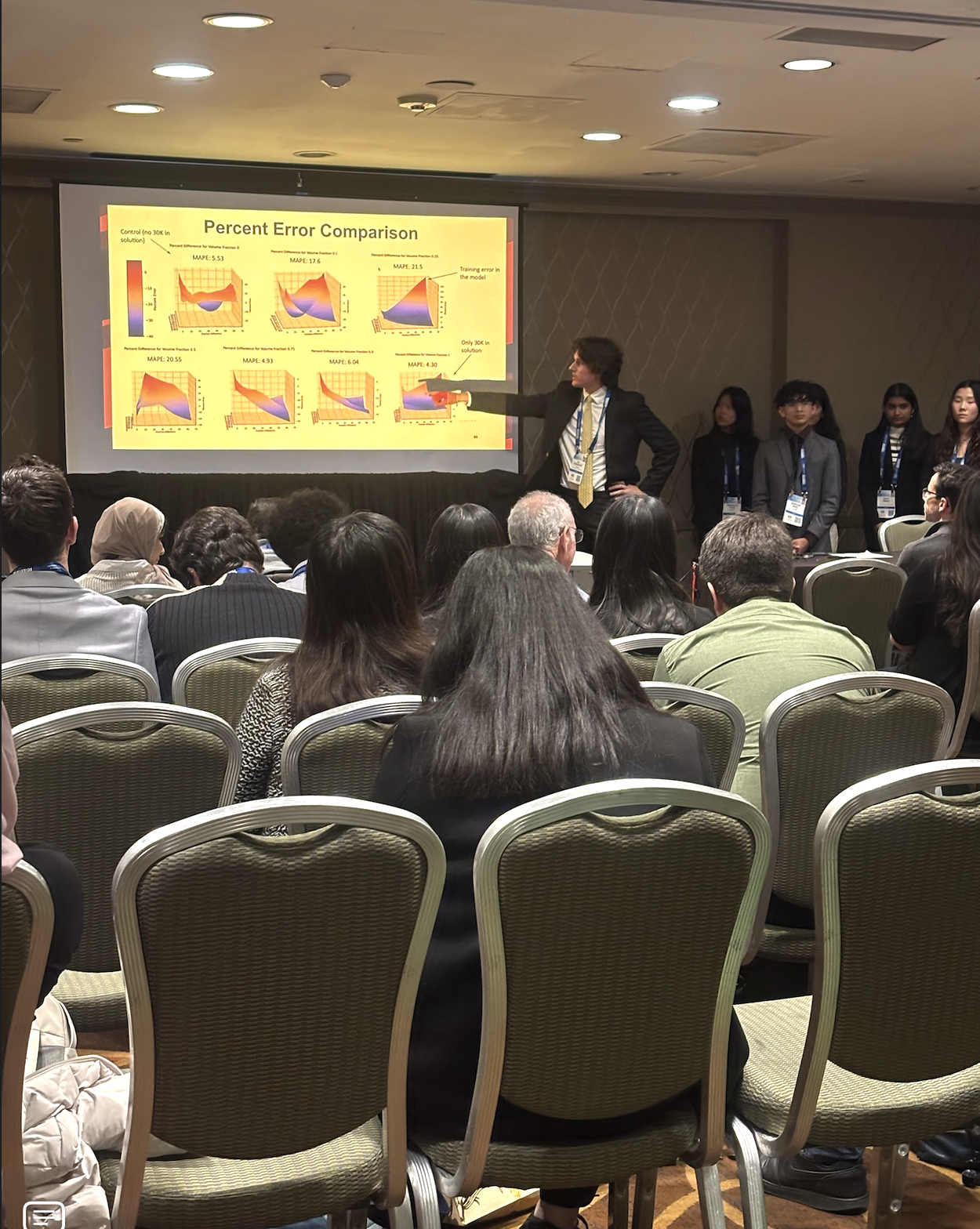
Abstract as submitted to the symposium.
We have presented our work as an oral presentation at the Materials Research Society fall meeting on 12/4. See our abstract below:
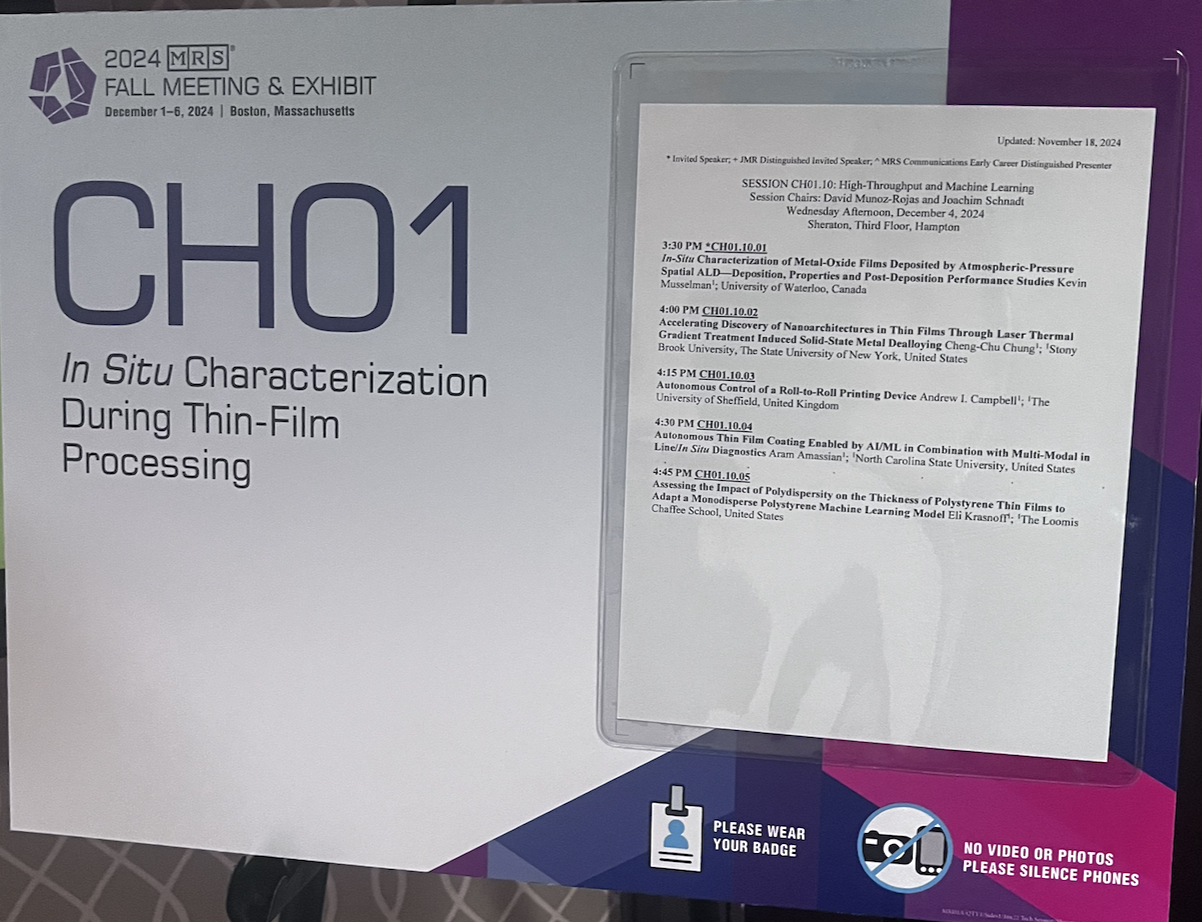
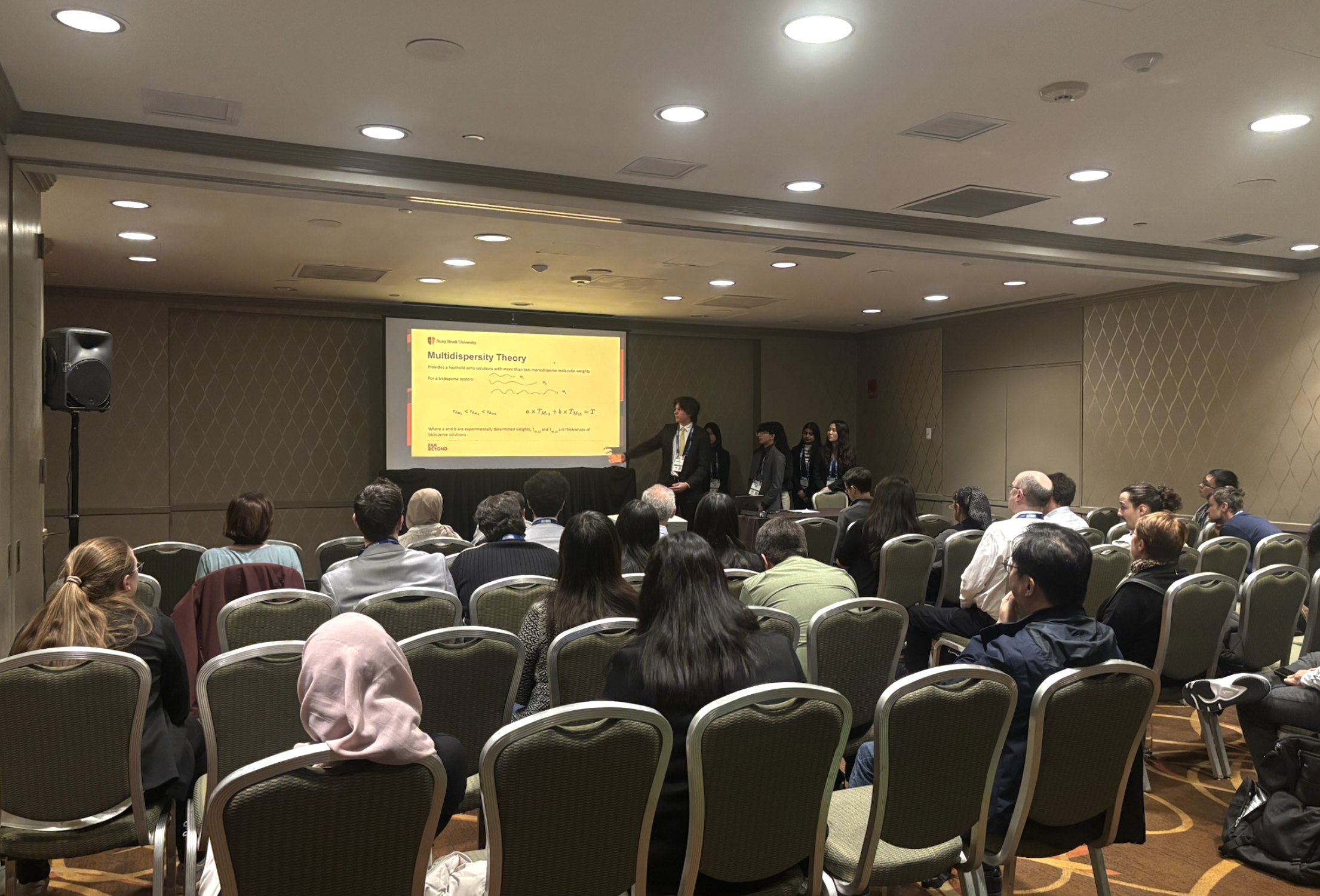
We have been accepted for oral presentation at the American Physical Society, and we are in process of submitting our manuscript to Polymer Testing.
We have been invited to present on our work at a weekly meeting assembled by Dr. Yuefan Deng. See his work here.
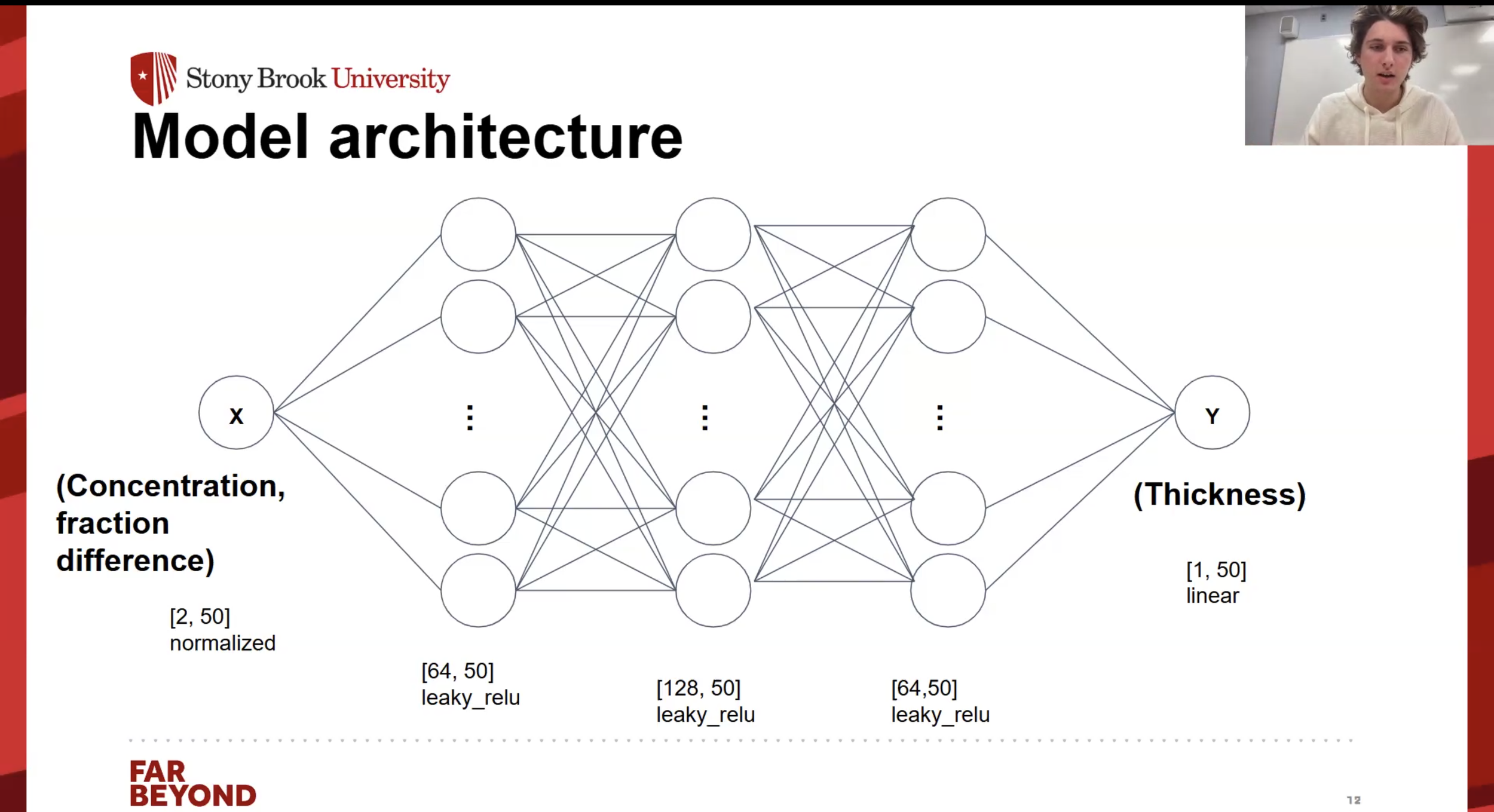
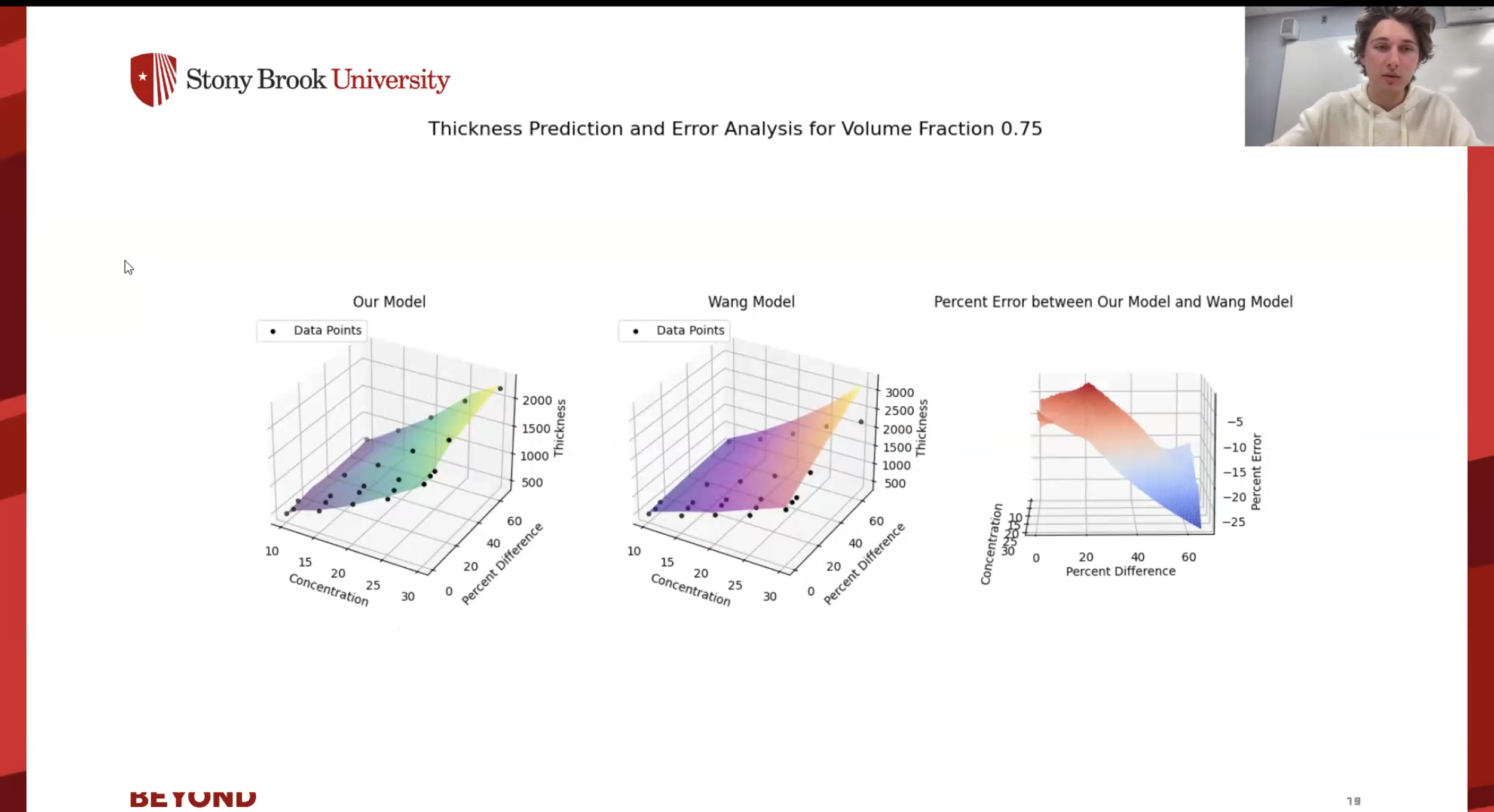
Yuefan Deng. Bio linked to photo.